Three Perspectives on Generative AI in Elections
Justin Hendrix / Oct 27, 2024Audio of this conversation is available via your favorite podcast service.
In recent months on the Tech Policy Press podcast, we’ve repeatedly come back to the question of how generative AI is affecting democracy and elections. Last week, I spoke to three researchers who recently published projects looking at the intersection of generative AI with elections around the world, including:
- Samuel Woolley, Dietrich Chair of Disinformation Studies at the University of Pittsburgh and one of the authors of a set of studies titled Generative Artificial Intelligence and Elections;
- Lindsay Gorman, Managing Director and Senior Fellow of the Technology Program at the German Marshall Fund of the United States and an author of a report and online resource titled Spitting Images: Tracking Deepfakes and Generative AI in Elections; and
- Scott Babwah Brennen, Director of the NYU Center on Technology Policy and one of the authors of a deep dive into the literature on the effectiveness of AI content labels and another on the efficacy of recent US state legislation requiring labels on political ads that use generative AI.
What follows is a lightly edited transcript of the discussion.
Samuel Woolley:
My name is Samuel Woolley. I am the Dietrich Chair of Disinformation Studies at the University of Pittsburgh, where I am also just starting a new research lab called CTRL, which stands for the Communication Technology Research Lab.
Lindsay Gorman:
I'm Lindsay Gorman. I'm the managing director and senior fellow of the technology program at the German Marshall Fund of the United States.
Scott Babwah Brennen:
I'm Scott Babwah Brennen, and I'm director of the Center on Technology Policy at NYU.
Justin Hendrix:
I'm so excited to have the three of you on today to talk about generative AI and elections. Each of you have come at this question from a different perspective just in recent days and weeks, having released projects that look at different angles on the question of how generative AI is intersecting with elections.
Lindsay, I want to start with you. You've released this tracker, Spitting Images: Tracking Deepfakes and Generative AI in Elections, an interactive tracker that's looking at a variety of different types of AI artifacts, from audio to images, to video, to different multimodal types of media.
You're looking at some of the bigger incidents that have happened around the world. I can scroll around this thing, spin the globe, and see what's been happening over the last several weeks and months. How would you describe this project and what are some of the key things that you've observed?
Lindsay Gorman:
Yeah. Thanks so much, Justin. I think our ... Let's start with our motivation for the project, which was that about a year ago, we recognized that there is all this hype and angst and agita about how AI was going to impact elections around the world, or in 2024, it's said to be the largest election year in history, with about half the global population heading to the polls or headed to the polls already. It was in this head-on collision with the development of generative AI and the ability to really have a democratized capacity to create realistic fake images and video in addition to obviously generative text, as we've all played around with ChatGPT.
And so, there was this sense, I think, in the policymaker community that this was going to be a huge problem. What are we going to do about it? And so, with Spitting Images where the title, I imagine, these politicians, the generative AI images of these politicians spitting at each other or something, our aim was really to categorize how are these deepfakes actually being used in elections around the world? Are they proximal to the elections? Are they coming right up on the eve or in the months and weeks before? What types of AI is actually being used in the real world?
So that's why we categorize these instances and we've mapped around 133 instances in elections around the world, across 30 countries in five continents. We're trying to understand, what are we really looking at so that as policymakers, as researchers, we can think about what do we really have to be concerned about here?
And so, some of the key findings that we found originally in this first look at the data, now almost at the end of 2024, is firstly that these things are inordinately prevalent. Over a third of elections that have been held since we started tracking this back in 2023 have actually featured a major deepfake campaign that's gotten significant traction to be picked up by major media.
The tools also have varied, but actually audio deepfakes are the most popular tool in our dataset. So 69% of the instances we track actually just feature an audio deepfake, AI-generated audio. In comparison with 55 that feature AI-generated video, only 20% have AI-generated images.
So I think what that says from a policymaker perspective is that we really need to be paying attention to audio. I think we all remember the robocall of President Biden in New Hampshire from months ago, encouraging voters not to go to the polls. That is overwhelmingly the type of deepfake that we're seeing in elections around the world.
Then I think one last point that's really come out of this broad look globally is that there isn't really one mode of how candidates or how opposition parties are using deepfakes in elections. In some cases, there seems to be an intent to deceive, but in other cases candidates are just using these tools for artistic expression, to promote a certain image of themselves.
Now-President Claudia Sheinbaum in Mexico used a deepfake image of herself in the campaign. It had six fingers. But we're seeing also in Argentina, we saw instances of candidates creating AI-generated posters.
So this isn't just the deceptive, but also the artistic expression. I think we'll see how it develops and how this technology continues to be used in elections around the world.
Justin Hendrix:
Your mention of Mexico's perhaps a good place to transition to Sam and ask about your project, which also has given us a world tour of generative AI and its use in elections in the US, Europe, India, Mexico, and South Africa. Don't know if you want to pick up there on Mexico, Sam. Tell us a little bit about what you've learned looking around the world.
Samuel Woolley:
Sure. So these are a set of comparative studies written by folks either in-country with expertise in these areas, or people that are from those places with other expertise on those places and spaces.
Yeah, Mexico is a particularly interesting case. There was some evidence there from the researchers that conducted the report that there was deepfakes of high-profile politicians flowing. Also, the idea, the question of whether or not some of what we were seeing amounted to genuine disinformation or manipulation or whether it was satire or humor or things like that. I know this is a perennial question that comes up. My own perspective is that it can be both and that it can still be damaging even if it's satirical or funny use of disinformation given the polarized environments that we live in.
Across all of the cases that we analyzed that really reached across the globe, we found that generative AI, and AI more broadly, are becoming important tools in the political campaigner's toolkit and in a political communication toolkit. But that a lot of the use, especially use in terms of external communications, is still speculative. It's still a space where people are playing around with the tech. They're seeing how they can use it.
Listeners will be familiar probably with the articles that have come out recently saying that generative AI and its impact on elections has fallen short of what the expectations or hype was this electoral cycle. What I can say is that while I understand where those arguments are coming from, I think that they're failing to take the balanced approach that we see actually playing out across the country case studies, meaning that in South Africa, in Mexico, in India, US, and Europe, while it's true that perhaps generative AI is not the most used tool or the most efficacious tool, it's also true that it is becoming normalized for use in politics and that the potential continues to grow.
Oftentimes I think that what we see from these case studies is that there's a focus on things like AI-generated deepfakes or AI-generated audio, but also we oftentimes overlook the importance of broader uses of AI, particularly on the back end for data analytics and particularly for micro-targeting of particular marginalized communities across all of these cases.
And so, while I think about it as like a car, we look above the hood and we can see the car and see how sleek it is and talk about all these things as we do with generative AI, but really we need to look under the hood to understand what's going on on the back end as well. That's a big finding from a lot of these reports.
Justin Hendrix:
Scott, I want to bring you in. You've issued a pair of reports in the past couple of weeks, one on a question that's specific. Will AI content labels work? So I want to find out a little bit about that. Then one specifically looking at perhaps more US context, looking at state-required AI disclaimers on political ads. I think you're responding generally to the enthusiasm among policymakers to try to answer the potential threat of generative AI with labels and disclaimers and disclosures. I'd love to know what you've learned about whether any of that stuff will help.
Scott Babwah Brennen:
Yeah. So you're right. We did take this grandiose approach where ... Seeking to answer, will AI content labels work? And so, I guess why don't I start with the experiment, which is a bit narrower in scope?
So far, something like 20 states have passed laws regulating in one way or another generative AI in political ads. Most of these laws required disclaimers or labels on certain uses of generative AI in political ads or other political communication. Yet, as far as I know, there have been no empirical analyses of how effective these interventions are, what impact they might have on campaigns.
So in the experiment that we designed, we created some of our own political disinformation. We created a pair of fake advertisements, and then that included generative AI in different ways. Then tested out the labels that are required now by Michigan and by Florida. Then we also created a bunch of conditions where it seems like the ads are coming from Republicans or coming from Democrats or from ... There's no clear political affiliation.
Basically we saw four key findings. First, AI labels hurt the candidates that use generative AI, and even resulted in what we're calling a backfire effect for those candidates that made attack ads. What we mean by this is that in the conditions that had labels, respondents reported having a reduced opinion of the candidate in terms of trustworthiness or appeal, a reduced perception of the accuracy of the ad, and a reduced intention to share it or like it if it were on social media.
As far as the backfire effect, what that means is that basically in the attack ad, we have the candidate who made the ad and the candidate who is being attacked. In the conditions without labels, they were equally rated in terms of trustworthiness or appeal. In the conditions with the labels, the attacker was rated ... The appeal and the trustworthiness of the attacker dropped, but there was no effect on the candidate being attacked. So we saw this effect just on the candidate who created the ad, not on the candidate who just appeared in it.
I can go through the other findings really quickly, because I'm sure we'll want to talk more about some of these. But, second, this effect that we're seeing seems to be a result of respondents lowering their assessment of members of their own party or of nonpartisan candidates rather than members of the opposite party. This says actually the exact opposite of what we hypothesized, but makes sense, right? It's like we all have low opinion of opposition candidates.
Third, the label effects were small. We saw significant effects, but they were generally small. This might in part be a result of the fact that many viewers didn't actually notice the labels. This really underscores that design and wording actually really matter here when you're creating labels.
Then, finally, we asked respondents about their opinions about different policy interventions, the ones that are now being adopted by states. Respondents were least supportive of the policy approach that is enacted by most of the states that's requiring labels only on deceptive uses of AI in political ads. Some of the other options are banning all generative AI in political ads or requiring labels on all uses.
Justin Hendrix:
I want to get a little into some of the specifics and some of the things that people are concerned about. I also want to talk a little bit about some of the concerns and recommendations you have here for policymakers, especially in the United States, the concern around whether content-based restrictions, labels, things of that nature might trigger strict scrutiny under the First Amendment. So we can talk about that a little bit in the domestic context.
But given I've got three researchers on the line and we're talking about this wave of elections, we're talking about generative AI as a new technology that's being introduced around the world, almost instantaneously across all these various national contexts, how in the world do we study this phenomenon? Do we have the data? Do we have the prevalent information that we need? How are we set up as, I suppose, scientists to look at these questions?
I don't know who to quite put that to other than just ask each of you whether you feel like we have the scaffolding in place to really get at this broader question here of whether generative AI is having a meaningful impact on democratic processes. Go ahead, Sam.
Samuel Woolley:
It's a big question, of course, but I'm happy to take a swing at it. I think that, as with many other phenomena, it's true that there is no one approach that is going to give us all of the things that we need to answer this question. No one discipline is going to do that work, nor quantitative computational or qualitative researchers alone are going to be able to do this.
I think a lot of the critiques we've seen that have come out recently saying that there is a lack of impact of GenAI on electoral contests. For instance, in Europe, the stuff from the Turing Institute and stuff, they come from a particular scholarly position. They come from a particular way of studying these sorts of phenomena. While I respect that work, I also think that there are other ways of looking at this that reveal greater impact and that reveal the limitations of those kinds of studies and of broader studies.
So as with everything, you'll hear academics talk about when it comes to tech and studying the impacts of tech upon society, you're going to hear people say there's a lack of access to data. The same is true here as it has been with social media.
So it means that while we have some access to data and, for instance, in the Turing study and others, we depend upon news reports, or we see scholars depending upon news reports, of the use of GenAI in electoral context, those things have major shortcomings. So journalists will be the first to tell you that they aren't able to report on every single event and that they make decisions about events to report upon when it comes to GenAI or anything else.
I was talking to someone recently, they were saying, for instance, take for instance the ongoing conflict in Israel-Palestine. Every day a journalist that's on the ground there has to make really difficult decisions about what they're going to report upon. The same thing is true during an electoral contest. And so, we're relying upon news reports to tell us what's going on with GenAI, as imperfect in that of itself.
The other thing I think that we need to understand is that studying this stuff qualitatively can be really useful because it gives us an understanding of the phenomena in depth. Who's using it? How are they using it? Why are they using it? What are their intended outcomes?
We wrote a piece at Center for Media Engagement at UT Austin where I was prior to joining the faculty at Pitt, where we talked to 25 or so political consultants who were working on US campaigns and who were using GenAI in attempts to reach the electorate. Unanimously across the board, those folks said, basically, we need more study of this stuff. It didn't matter whether they were Republicans or Democrats. They also unanimously said they were terrified by the lack of guardrails in this space.
I think that one other area that's burgeoning for study beyond just understanding the impacts is understanding how we develop early policy to prevent some of the biggest misuses of this technology, despite the fact that the research is probably going to take a decade to really concretize, just as it's still taking time to concretize our understandings of the impact of things like disinformation upon voting processes and stuff like that.
I think that sometimes ... And this is the last thing I'll say, that sometimes with scholarship, we rely on scholarship to be able to tell us now what the impacts are. But any scientist will tell you ... That's worth their salt, they'll tell you it takes a long time to actually figure out what the impacts are. If we wait to know precisely what the impacts are for two decades or three decades, we will be in a really bad position because the capacity of generative AI for manipulation is absolutely there and it's already being realized across the world.
Justin Hendrix:
Lindsay, maybe I'll ask you to pick up where Sam just left off. Even just looking at some of your findings, there's a sense, a little bit, of a gathering pace of the use of these things in elections that ... You point out there's way more instances even recently in the US than there were in some of the elections that happened earlier in the year. I assume that has something to do just with the propagation of the software.
Lindsay Gorman:
Yeah. I think it's indisputable that the use is on an upward trend. I agree with a lot of what Sam said, that as policymakers, we also need to deal with the here and now and some of these questions. What impacts an election? What advances a particular view of a candidate? What sticks in people's minds are notoriously difficult to really understand and to really capture. This is something we've seen with disinformation since time immemorial, really. The age-old question is does this piece of content really have an impact on voter sentiment?
I think some of the researchers on this call have done great work in this space, but I don't think we have a definitive way of determining that even as, anecdotally, we can see that narrative spread through some of the survey research and polls. We can see voter sentiment and opinion, but just how much a particular piece of content contribute to that, I think, is nearly impossible to tell.
I think one area where there has been, really, I think a backsliding in terms of our ability to understand these questions is the access to the social media platforms and in terms of the access they provide researchers with just to study how content is spreading. And these private companies are on the front lines. This is not just a government problem. We've seen a lot of the legislation that Scott alluded to also, not just in the United States, but in the EU AI Act itself imposes transparency requirements on political AI, particularly around elections.
But it isn't just for governments to mandate this stuff. I think our social media companies have a big role in making sure that they are accurately labeling and quickly labeling content in a way that people actually see as opposed to a teeny footnote that people will ignore. So a lot of these tools, I think, are in our toolkit and it's just really about adopting them.
But very much agree with the point that we can't wait until we fully understand the full scope or the impact before we take action, because it is increasing. As you mentioned, in our findings, we saw there were nine times as many deepfake campaigns in the US elections as the UK. Obviously there are different population sizes there. But just, I think, the scope of their use is not something we saw two years ago, four years ago.
The muddying of the information space is not unique to generative AI. Clearly this has been the case with disinformation and all kinds of texts. Anyone can say anything. But I think what is new is this idea that we can't trust what we see anymore with images and video content that's generated.
Right now a lot of them are not incredibly sophisticated. You can usually tell when something's been manipulated. But this technology is advancing and I think it's going to decrease our overall trust in the information environment, which is really important for democracies. Autocratic governments thrive when we can't tell fact from fiction. Here in democracies, we need it to select our political leaders. We need it to be able to hold politicians accountable.
We've even seen instances when our dataset that have been popularly shared of cases where politicians said, no, that wasn't real. That was actually AI. So I think it goes both ways when it comes to trusting the quality of information that we're viewing.
Justin Hendrix:
Maybe, Scott, I'll just toss it to you as well. It sounds like from your research that policymakers are also essentially fumbling forward, in some cases, making policies or even passing laws, some of which perhaps needed a bit more time in the legal consideration before perhaps they made it to a governor's desk. But it sounds like as well that we're missing some important data on how best to make policy here.
Scott Babwah Brennen:
Yeah, for sure. I should say, researchers, we're always going to say that we need more research, we need more data. I think that's part of the job. But you're absolutely right. Not only can we not wait until we fully understand the situation to act, but policymakers aren't waiting. They're rushing ahead and enacting laws.
You're right. In the reports we put out last week, we argued that given what little we know about the impact that design, that wording choice has just in the context of labels, we really could use a little bit more study on just how can we maximize the benefits and minimize the disadvantages, the costs of a particular piece of legislation? We know that word choices like manipulated or calling out specifically AI in a label, these things really matter. We really don't know, though, very well what a label really should look like. We want to give it the best chance of having significant impact.
I should say I don't have much else to add based on what Lindsay and Sam said, which I agree with almost entirely. But I do want to just underscore one point about the difficulty of assessing the direct effects of pieces of disinformation and the ... But that's absolutely true. It's also even more true that it's hard to ... That doesn't make sense. But it's also true that it's hard to assess the secondary effects.
So it's not only that we care about that piece of disinformation will convince someone that something that is not real is in fact real, but we care about the bigger effects that the accumulation of disinformation might have on [inaudible 00:25:53] institutions more generally, on people's engagements in politics. These things are incredibly difficult to really understand.
Justin Hendrix:
The issue there is often time horizons. We're talking about things that may change over time. It also strikes me that it's also about the fact that generative AI is introduced into a context. It's not just about the generative AI, it's really about where it propagates, which is on social media and, in some cases, through traditional media. I don't know if, Sam, you want to pick it up there.
Samuel Woolley:
Yeah. Just after listening to Lindsay and Scott talk, something that I thought of and that relates to what you're just saying, context matters, absolutely. It makes me think of Sareeta Amrute's research at Data & Society, along with her colleagues, that says perhaps one space we really need to be looking in our research is the majority world when we're trying to ascertain what the impacts of this kind of stuff are, because, as we saw with disinformation or as we saw with big data-oriented manipulation from the Cambridge Analyticas of the world prior to them being shipped over to the US, these kinds of things were being practiced in the majority world as a Petri dish to see what worked and what didn't by these political marketing organizations. The same is true. We're seeing a lot of this going on in places like India and Indonesia, across the Middle East.
And so, one thing I would hope is that researchers put a focus upon the majority world and try to understand where the impacts are there, because oftentimes in the United States and the UK and Europe, the people who are launching these kinds of campaigns are reluctant, or they realize that if they get caught there, the stakes are much higher.
Then the other thing I'll say is, on the question of the First Amendment here in the United States, I've said this in other spaces and I'll say it here, which is that I think what's illegal offline should be illegal online. It should be illegal to leverage generative AI to mislead people about how, when, or where to vote. It should be illegal to leverage generative AI to spread credible threats of violence.
But also I think that oftentimes we think about these kinds of questions as a dichotomy between either privacy and safety on the one hand and free speech on the other, and I reject that dichotomy. I don't like it. I think that it's like politicians and policymakers have to be able to walk and chew gum at the same time, and we have to learn how to prioritize all of those things. That's always been the case. That's why the Constitution and the Bill of Rights exist.
For some reason we've come into this space now where you have the Elon Musks of the world saying we need free speech at all costs. My response to that is the online ecosystem is not an open marketplace of ideas anymore. It's a completely flawed marketplace and garbage, and actually powerful organizations and individuals that use GenAI can leverage it to try to control public opinion in a very big way.
Scott Babwah Brennen:
I'll jump in there. Yeah, Sam, I agree with a lot of that. But I guess two things. First, unfortunately, we don't have a federal prohibition on disinformation about time, manner, place in election disinformation. Some of the states do have individual laws, and this is something that we've been calling for the center for years now, writing about how important that would be. It's funny, I think Obama as a senator actually introduced a bill back in the early 2000s that would outlaw it.
Yeah, I think whether or not we agree about what the law should be, I think we have to deal with the reality that the First Amendment is going to be a significant impediment to some of these new regulations or pieces of legislation. The way that the First Amendment is currently being interpreted basically precludes strict prohibitions on limits on misinformation as we're now seeing, for example, in the district court enjoining the recent California law requiring, I think, labels on political ads.
I think, Justin, this goes back to a point you made a few minutes ago about the need for policymakers to take a little bit more time to sort out how they can pass impactful legislation that is going to withstand legal scrutiny.
Lindsay Gorman:
Yeah, I agree with those points, but I also think that there are ways that we can apply our current legal frameworks here. We're not starting from zero. It's not like online threats are immune to some of the legal protections that we're accustomed to, that we're operating under in the offline world.
I think one example of this, and we talked about it earlier, this New Hampshire robocalls. The guy who essentially created those was indicted for a felony, for voter suppression and impersonation of a candidate as a misdemeanor. I think the FCC proposed a multi-million dollar fine for him.
So these things are not necessarily happening with impunity. I think it's very hard to determine. In a lot of cases, we found attribution of who's actually creating these things, and that's very much a bottleneck. But when that's possible, some of our laws can apply.
Scott Babwah Brennen:
Yeah, for sure. Unfortunately, the FCC has a limited jurisdiction. And so, it covers things like robocalls or broadcast TV, but doesn't oversee digital ... Like online advertising or online content. The FEC that is, I think, in the middle of the debate about where exactly their authority is, given the way that the FTCA was written, that if they have the ability to regulate this sort of deceptive content, and I think a member of the current commissioners don't believe that they do currently have that authority and are calling on Congress to expand their authority. I'm pretty skeptical that will actually happen. But as it is, I think there's limited chance that we're going to see [inaudible 00:32:27] enforcement [inaudible 00:32:32] the sort of deceptive content in digital media.
Samuel Woolley:
One thing I think we're not mentioning is that there's very much a politics to all of what we're discussing in this country right now, and it's unavoidable. Everyone knows about it. It's like the elephant in the room. These bodies that we're talking about themselves are political bodies in terms of who's put on these spaces and whether or not they actually can act based upon the makeup of the body or what they lack, like we saw during the Trump administration in terms of sitting members.
It remains true that there's this broad-scale, somehow, acceptance, particularly in the Republican Party, that any kind of suppression of speech on social media is tantamount to censorship when in fact Section 230 of the Communications Decency Act allows for exactly that. It allows social media companies as private entities to determine what they would like to have on their platforms and what they would not.
And so, there's this song and dance that I see going on right now in this space that is a little bit disconcerting, where we've almost let social media companies go back to the space again where they're trying to be just the pipes or a telephone company or something again when everyone knows that's absolutely not the case with how they actually operate.
Justin Hendrix:
So I want to draw this to a conclusion here, because we've gone now for our 35 minutes we planned. But I suppose what I want to do is maybe just ... Lindsay, you mentioned that this is the year of elections. We've still got a couple of very important elections ahead, including the one here in the United States, as I'm talking to you on October the 22nd. But what are you looking ahead to in 2025? What will be the key questions you'll want to ask on this subject in the year ahead? Maybe we can just go around and ask each of you to give a little look forward.
Lindsay Gorman:
Yeah, it's a great question. I think what we see with new technologies and new platforms is that there's often an experimentation phase. Then in some cases users coalesce on the best practices in how to use that technology.
And so, a separate work that we do is studying how candidates are using TikTok as a new platform for political and electoral outreach. We saw this in 2022 midterm elections in the US. It was very much in the experimentation phase, and now some of this is starting to coalesce.
And so, I think 2024, this year, we've very much seen this experimentation phase. We've seen everything from artistic expression to deceptive deepfakes. We've seen things shared openly by candidates. We've seen on one zanier example from just the last couple weeks, we had an instance of an AI bot participate in a debate in a Virginia House race created by a candidate to mimic his opponent.
So I think we've seen it all this year. I think with 2025, one of the questions is going to be, are there best practices for how to have impact with generative AI that will start to coalesce from different actors? Will it be when a deepfake is released in relation to an election? Will it be the medium that's used? Will it be an intent to deceive or an artistic expression? Then continuing to refine our sense, which is a very hard question, as we've discussed, on what the impact is.
I think one thing that Scott mentioned earlier in the program was this backfire effect. I think that's something we've seen already. One of the examples in our dataset is when Trump shared AI-generated images of Taylor Swift purporting to endorse him. Then it was just weeks later that in fact she cited, Taylor Swift cited, those AI-generated images when she was actually endorsing Harris. So talk about the ultimate backfire effect.
So I think these are the kinds of impact questions that we are just getting some hints of in 2024 and 2025, as this technology matures, as the use of it matures, we'll hope to get a better sense of.
Justin Hendrix:
Either of you want to go next? Final thought, what you're looking for in the future.
Scott Babwah Brennen:
Sure. I guess a couple things. One, at least in the US, I think, as we said earlier, there was a great deal of concern at the beginning of the year that we would see just a huge amount, a tidal wave of generative AI, deceptive generative AI, in electoral content. I don't think we've really seen that. We've seen plenty, believe me, and some of those are really concerning. But I think it could have been much worse than it has.
Certainly the next couple weeks, as we get in the last two weeks of the election, who knows what's going to happen, and afterwards. But one thing I'll be looking forward is how political consultants, especially the big firms, start embracing these tools a little bit more than they have.
My sense of talking to consultants is that they have actually been hesitant to really embrace these tools. I think there's a couple of reasons for that. Some have suggested it's like regulatory issues, like they're not sure [inaudible 00:38:17] regulated, but I think also it's still somewhat hard to use. I think when we start having vendors developing tools that make it really easy for campaigns or consultants to use, I think we'll really see a lot more.
The second is I think we're going to continue to see state laws on AI being considered and passed. This past year, there have been many bills considered on AI, and a handful have passed, especially in places California or in Colorado, we had the only comprehensive AI legislation. I wouldn't be at all surprised to see that same Colorado bill appear on dozens of states in the next year. And so, we'll see a really renewed continuation of the interest in the past and aggressive AI regulation.
Justin Hendrix:
Dr. Woolley, that leaves you with the last word.
Samuel Woolley:
I think my answer is that next year, in 2025, what I'll be looking at is the international context, really, probably focusing on the majority world, focusing on ... Insofar as I focus on the United States, focusing on the impact upon marginalized communities, the use of this tool, how communities themselves feel that they were impacted, like what they experienced and what can be done better through a process of co-learning.
2024 is not the biggest election year ever. But 2025, there's a number of elections. Argentina, Ecuador, Poland, just to name a few. I expect to continue to follow those elections and see what happens as these tools, like both Scott and Lindsay have said, become more concretized in their use by political consultants across the world.
Of course, I'm really hoping that we see some of the fantastic experimental social scientists and computational social scientists out there do more work to ascertain what the impacts of these kinds of technologies are. Not on just a primary level, but secondary and tertiary level as well. Yeah.
Justin Hendrix:
Some answers, many more questions, a lot of work to do for everybody in this community going forward. But, Lindsay, Sam, Scott, thank you very much for joining me.
Lindsay Gorman:
Great to be here.
Scott Babwah Brennen:
Yeah, thanks for having [inaudible 00:40:55].
Justin Hendrix:
Cool. Okay. I'll press stop.
Authors
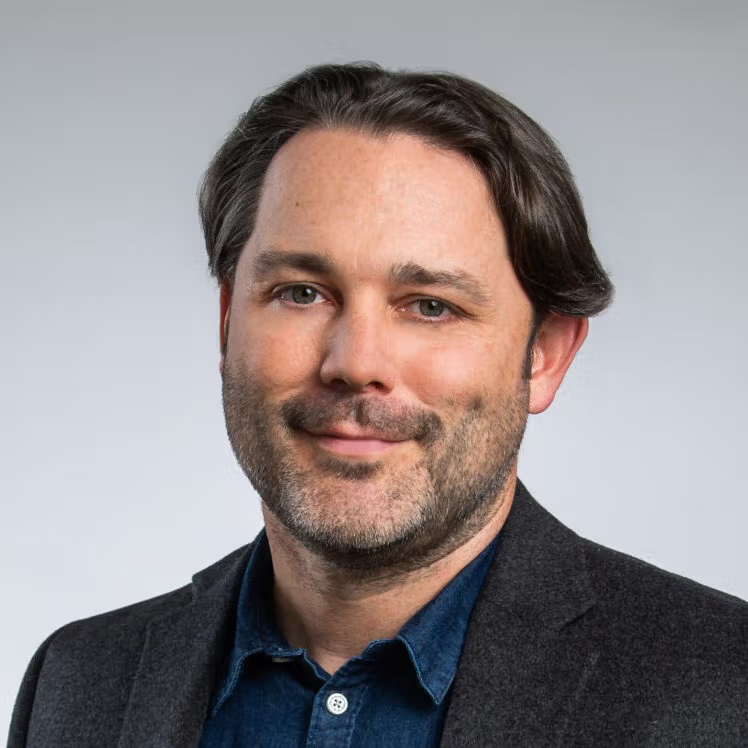